Upcoming Events
CSE Faculty Candidate Seminar - Qi Tang
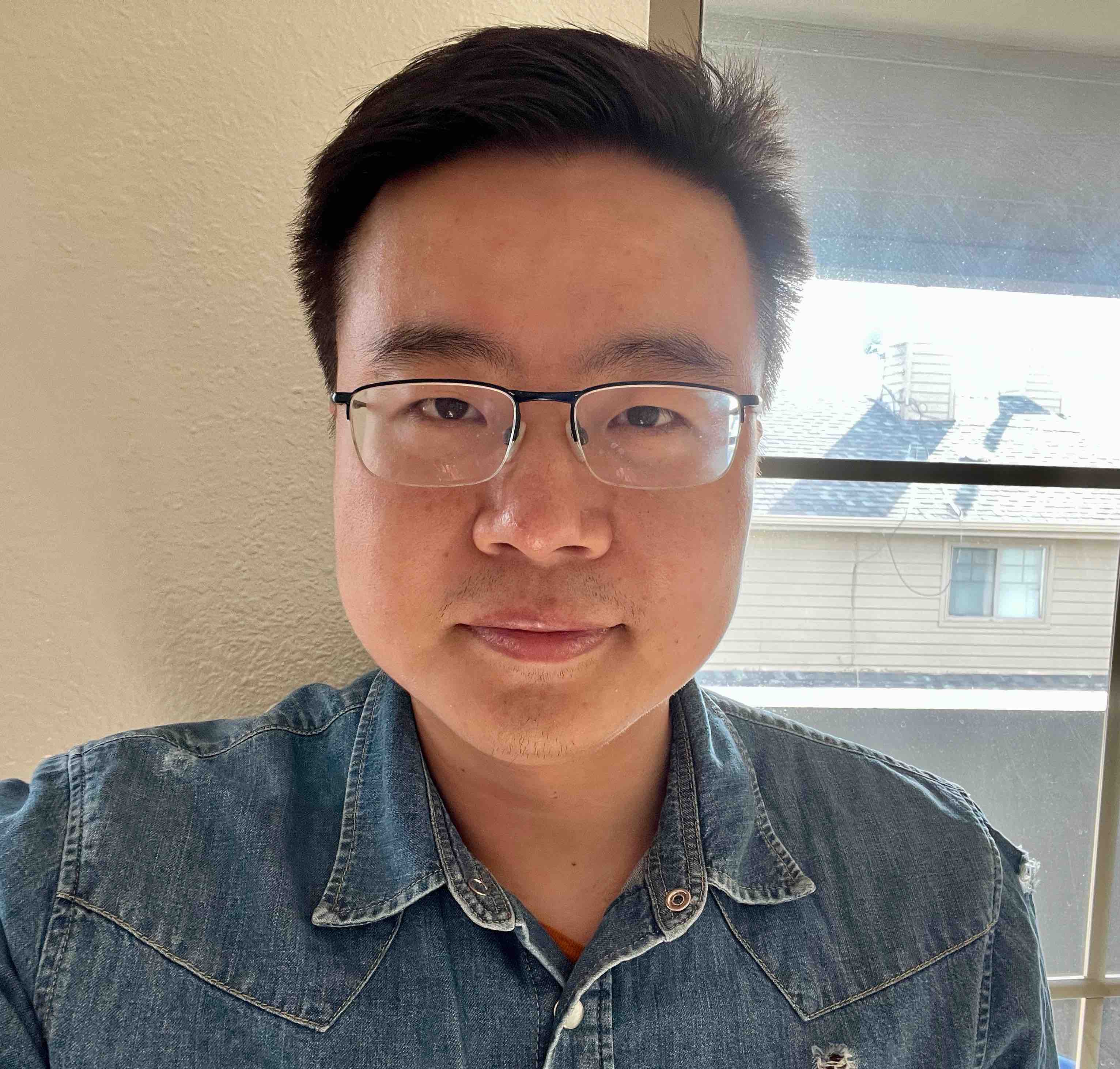
Name: Qi Tang, staff scientist at Los Alamos National Laboratory
Date: Thursday, February 15, 2024 at 11:00 am
Location: Coda Building, First Floor, Room 114 (Google Maps link)
Link: The recording of this in-person seminar will be uploaded to CSE's MediaSpace
Title: Bridging Scales in Fusion Plasmas through Scalable Numerical Algorithms and Structure-Preserving Machine Learning
Abstract: Fusion plasmas exhibit extreme ranges of scales and high dimensionality, placing high-fidelity models well beyond the reach of current algorithms and computers. In this talk, I will discuss two distinct algorithms capable of bridging a wide range of length and time scales in plasma science.
The first part focuses on the magnetohydrodynamics (MHD) equations that are an important fluid model to describe large scale instabilities in fusion devices. However, efficient numerical solution methods for MHD are challenging due to multiscale nature, strong hyperbolic phenomena, and nonlinearity. I will discuss the development of a high order stabilized finite element solver to overcome these challenges. Bridging temporal scale is achieved through a fully implicit solver with physics-based preconditioning, while the spatial scale separation is overcome via an implicit AMR algorithm. An island coalescence problem will be presented to show the advantages of resolving scales.
The second part explores structure-preserving machine learning for singularly perturbed dynamical systems. A powerful and well-known tool to address these systems is the Fenichel normal form, which significantly simplifies fast dynamics near slow manifolds. I will discuss a novel way of its realization using structure-preserving machine learning. Specifically, a fast-slow neural network is proposed, enforcing the existence of a trainable, attractive invariant slow manifold as a hard constraint. Analytical representation of the slow manifold enables efficient integration on the slow time scale and significantly improves prediction accuracy beyond training time horizon. To illustrate the power of FSNN, I will show a fusion-related example that models radiation reaction of electrons in a tokamak.
Bio: Qi Tang is a Staff Scientist in the Applied Mathematics and Plasma Physics Group in the Theoretical Division at Los Alamos National Laboratory (LANL). He received his Ph.D. in applied math from Michigan State University in 2015 and BS in math & applied math from Zhejiang University in 2010. Before joining LANL in 2018 he was the Eliza Ricketts Foundation Postdoctoral Fellow at Rensselaer Polytechnic Institute. His research interests include: MHD, kinetic modeling, scalable numerical algorithms, multi-physics and multi-scale problems, and scientific machine learning. His current research is supported by DOE Office of Science ASCR and FES programs and the LANL LDRD program.
Event Details
Media Contact
Mary High
mhigh7@gatech.edu
EVENTS BY SCHOOL & CENTER
School of Computational Science and Engineering
School of Interactive Computing
School of Cybersecurity and Privacy
Algorithms and Randomness Center (ARC)
Center for 21st Century Universities (C21U)
Center for Deliberate Innovation (CDI)
Center for Experimental Research in Computer Systems (CERCS)
Center for Research into Novel Computing Hierarchies (CRNCH)
Constellations Center for Equity in Computing
Institute for People and Technology (IPAT)
Institute for Robotics and Intelligent Machines (IRIM)